초청강연
8월 27일(목요일) 초청강연 | |
10:00 - 11:30 | 황승원(연세대학교): Knowledge for Language Understanding |
최윤재(KAIST): Deep Learning for Healthcare: Interpretability & Scalability | |
13:30 - 15:00 | 신기정(KAIST): Large-Scale Graph Mining |
윤세영(KAIST): Optimal Sampling and Clustering in the Stochastic Block Model | |
15:30 - 17:00 | 옥정슬(POSTECH): Fundamental Limit of Meta Reinforcement Learning |
8월 28일(금요일) 초청강연 | |
10:00 - 11:30 | 임성빈(UNIST): Learning and Control under Uncertainty |
박종화(서울대학교): AI 와 감성적 피아노 연주/Expressive AI Pianist | |
15:30 - 17:00 | 문태섭(성균관대): Continual Learning with Node-Importance based Adaptive Regualization |
연사 및 세션소개
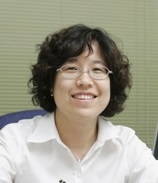
Knowledge for Modeling and Training for NLP Tasks
황승원(연세대학교)
본 발표에서는 언어 모델의 구축과 훈련에 지식을 활용하는 연구 사례에 대해 연세대학교 Data Intelligence 연구실의 최근 2년 연구를 바탕으로 소개한다. 관련 주제의 보다 자세한 정보는 http://dilab.yonsei.ac.kr/~swhwang에 있다.
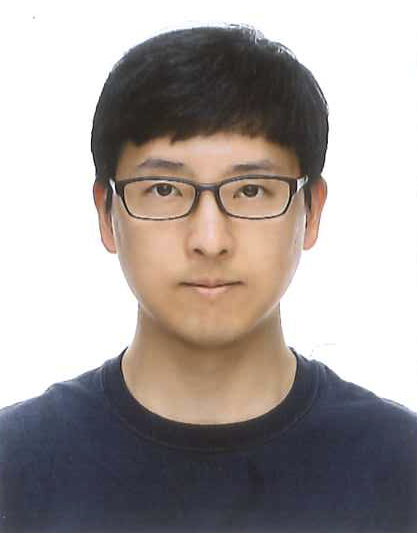
Deep Learning for Healthcare: Interpretability & Scalability
최윤재(KAIST)
Deep learning provides unprecedented opportunity to revolutionize healthcare thanks to the large volume of electronic health records. Although deep learning has demonstrated state-of-the-art predictive performance for various diseases, we must consider other practical aspects of computational healthcare as well, which are interpretability and scalability. In this talk, we will first briefly study how to handle electronic health records with neural networks, then address the two issues in more detail.
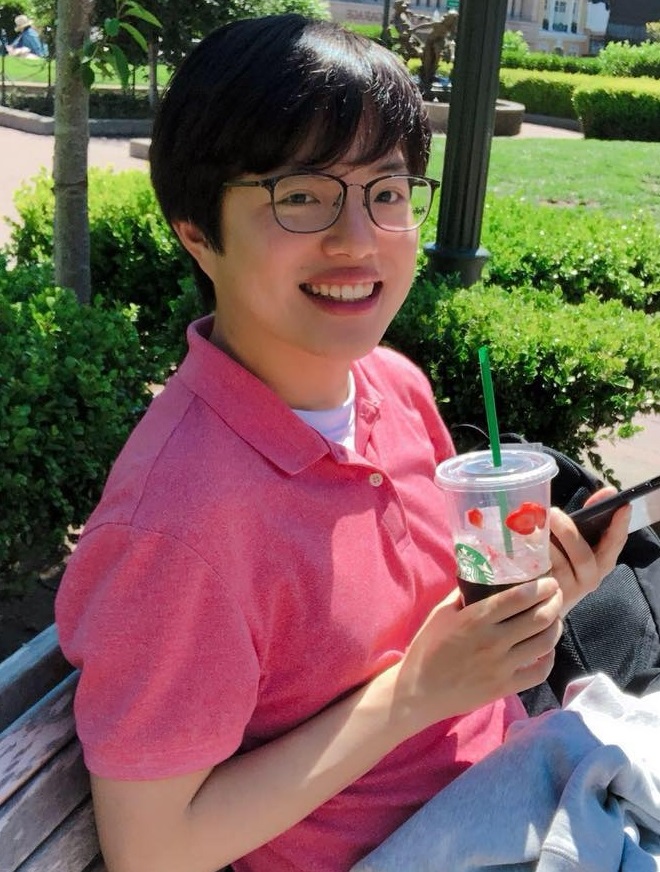
Large Scale Graph Mining
신기정(KAIST)
웹, 소셜 미디어, 리뷰 데이터 등 어디서든 쉽게 그래프 데이터를 찾아볼 수 있습니다. 그중 많은 데이터는 테라바이트 혹은 그 이상의 대용량이고, 실시간으로 변화하는 특성을 갖습니다. 또한, 많은 부가 정보를 포함하고 있습니다. 본 발표에서는 이러한 대용량의 동적인 그래프 데이터를 분석하고 활용하기 위한 도구들을 소개합니다. 특히, 데이터를 요약 및 압축하는 방법과 이상점을 탐지하는 방법을 중점적으로 소개할 예정입니다. 소개될 알고리즘들은 샘플링과 분산 컴퓨팅을 활용하여 수 테라바이트 이상의 대용량 데이터에 적용할 수 있도록 설계되었습니다. 또한, 소셜 미디어의 가짜 영향력자 탐색, 가짜 리뷰 탐색, 네트워크 공격 탐색 등 다양한 분야에 활용될 수 있습니다.
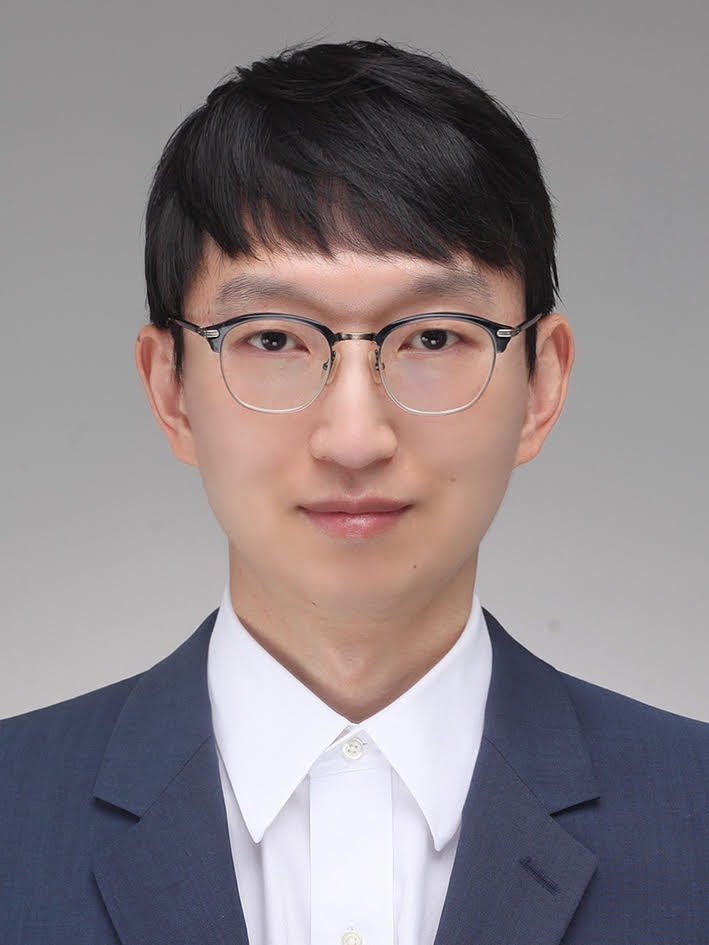
Optimal Sampling and Clustering in the Stochastic Block Model
윤세영(KAIST)
This work investigates the design of joint adaptive sampling and clustering algorithms in networks whose structure follows the celebrated Stochastic Block Model (SBM). To extract hidden clusters, the interaction between edges (pairs of nodes) may be sampled sequentially, in an adaptive manner. After gathering samples, the learner returns cluster estimates. We derive information-theoretical upper bounds on the cluster recovery rate. These bounds actually reveal the optimal sequential edge sampling strategy, and interestingly, the latter does not depend on the sampling budget, but on the parameters of the SBM only. We devise a joint sampling and clustering algorithm matching the recovery rate upper bounds. The algorithm initially uses a fraction of the sampling budget to estimate the SBM parameters, and to learn the optimal sampling strategy. This strategy then guides the remaining sampling process, which confers the optimality of the algorithm. We show both analytically and numerically that adaptive edge sampling yields important improvements over random sampling (traditionally used in the SBM analysis). For example, we prove that adaptive sampling significantly enlarges the region of the SBM parameters where asymptotically exact cluster recovery is feasible.
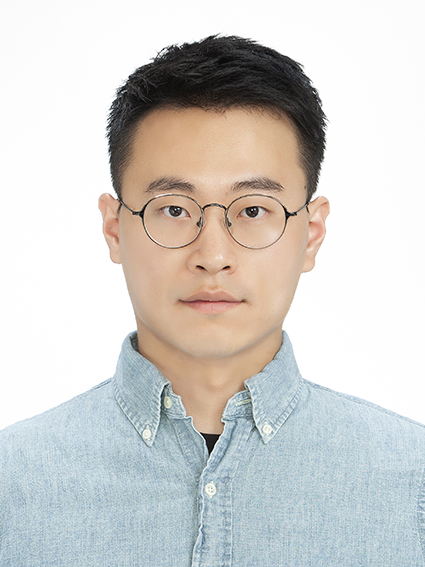
Fundamental Limit of Meta Reinforcement Learning
옥정슬(POSTECH)
Meta reinforcement learning is a framework which extracts and exploits the underlying structure of tasks to reduce learning cost. This is a promising approach to build scalable RL algorithms for practical systems with huge state and action spaces. In this talk, we present the fundamental limit on the benefit from optimally exploiting such structures, and an algorithm which achieves the limit asymptotically. Our analysis also shows that some continuity structure can reduce the learning cost significantly and provide scale-free cost. We conclude the talk with interesting primitive results on the learnability of structures in the transfer learning set-up.
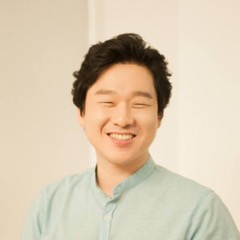
임성빈(UNIST)
ertainty is inevitable elements in learning and control problems. In this lecture, we will talk about the four types of incomplete data problem and their learning-based solution with uncertainty estimation.
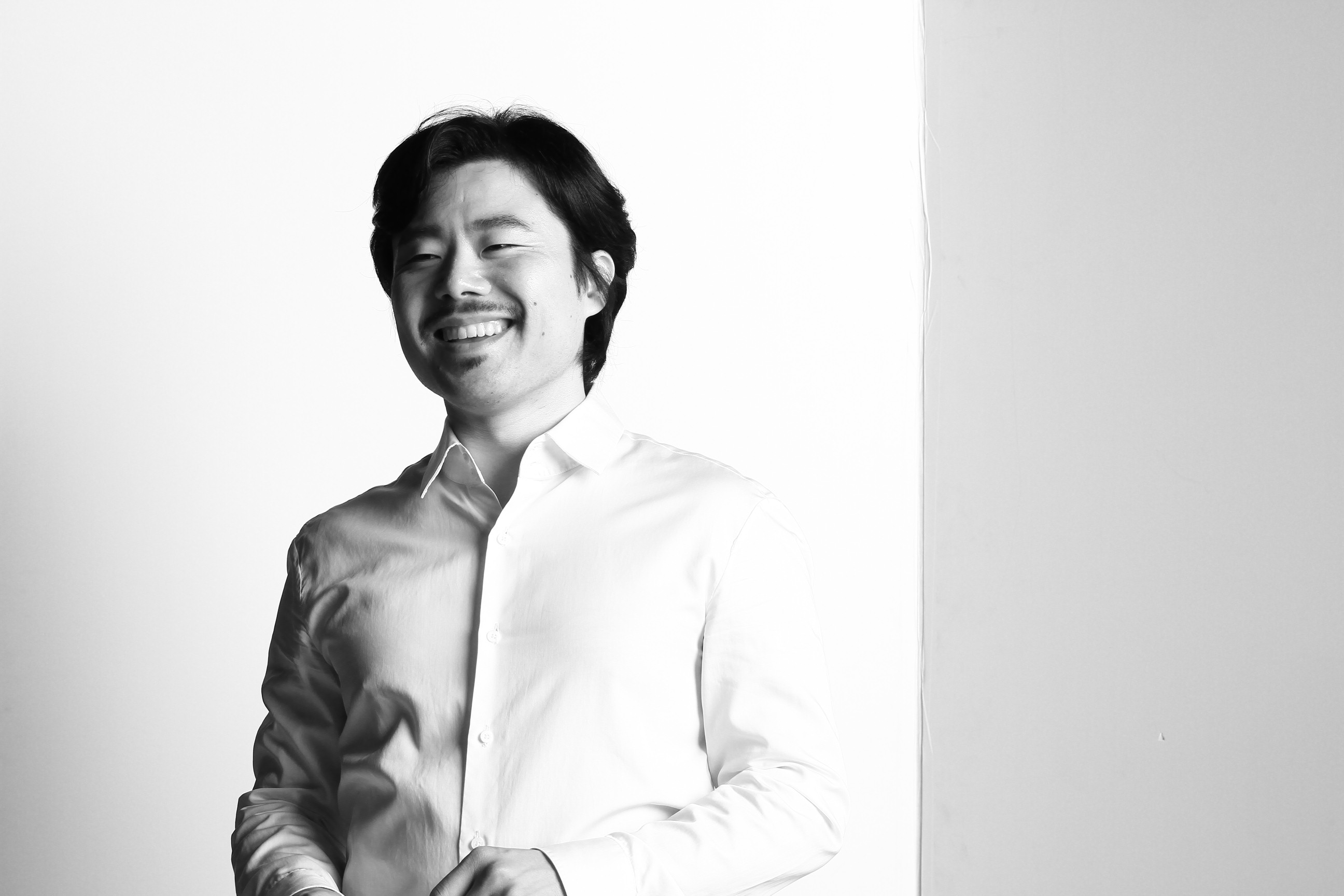
AI 와 감성적 피아노 연주/Expressive AI Pianist
박종화(서울대)
음악계에서도 AI 연구가 활발하다. 인간의 영역이라고 인지되고 있는 창의적 활동에도 AI가 automation을 가져올 수 있을까? 수행중인 감성적 AI 피아노 연구를 통해 미래의 음악 생태계를 살펴보고자 한다. AI has long been associated with automation of repeated tasks and tool sets that enable people to take advantage of computational power of machines. This work explores different applications in which AI can be exploited. Music and art, long considered a unique domain of human creativity, are being disrupted by algorithms. From algo-powered paintings to media art works that depend on current technologies, the boundary in the definition of creativity is being challenged. Expressive AI pianist explores the potential of machine-powered piano performance as a human interpreter would. The process regarding how machines extract information from a score and translate it into expressive performance on an instrument will be presented in this session.
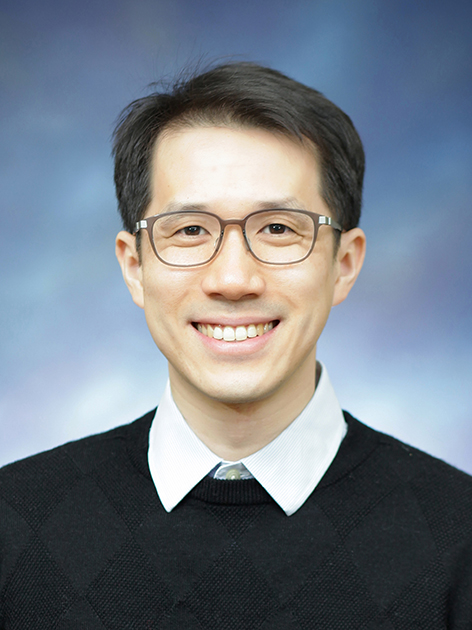
Continual Learning with Node-Importance based Adaptive Regualization
문태섭(성균관대학교)
Continual learning, also referred to as lifelong learning, is a long standing open problem in machine learning, in which the training data is given sequentially in a form divided into the groups of tasks. The goal of continual learning is to overcome the fundamental trade-off: the stability-plasticity dilemma. In this talk, I will describe two of my recent work on regularization-based continual learning, one based on Bayesian and the other based on optimization principle, that attempt to efficiently overcome the catastrophic forgetting via assigning importance to the nodes of a neural network, rather than the weights as in many recent baselines. As a result, I will show the proposed schemes convincingly outperform the state-of-the-arts in several benchmarks, with using orders of magnitude less memory for storing the regularization parameters. This is a joint work with my students, Hongjoon Ahn, Sungmin Cha, Sangwon Jung, and Donggyu Lee.