8월 16일 (화요일) | |
10:00 - 11:00 |
|
11:45 - 12:30 |
|
13:30 - 14:15 |
|
14:15 - 15:00 |
|
15:30 - 16:15 |
|
16:15 - 17:00 |
|
8월 17일 (수요일) | |
09:30 - 10:15 |
|
10:30 - 11:15 |
|
11:15 - 12:00 |
|
기조연설:
How To Scale Academic Research to Disruptive Innovation (60분)
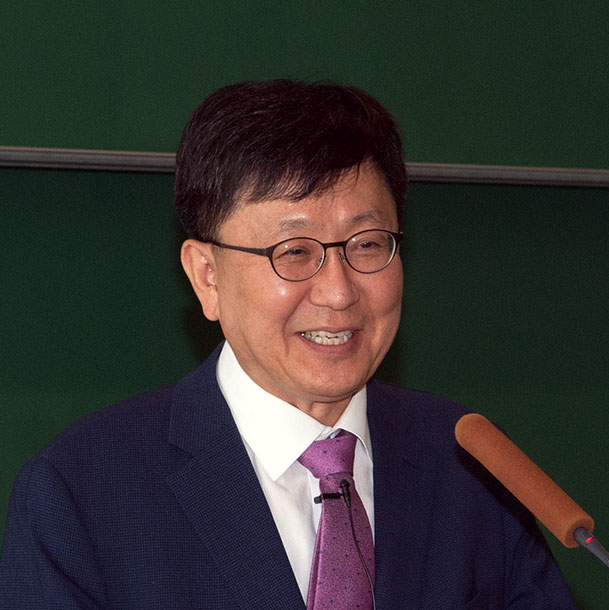
차상균 교수
(서울대학교)
Biography | |
---|---|
2022 | 삼성호암상 공학상 수상 |
2020-현재 | 서울대학교, 데이터사이언스 대학원장 |
2014-2019 | 서울대학교, 빅데이터연구원 초대 원장 |
2014 | 국가정보화 유공 근정포장 수상 |
2005-2014 | Founding Chief Architects, SAP HANA |
2000-2005 | Founder, Transact In Memory Inc., Silicon Valley Start up |
1991 | Ph.D. Database and AI, Stanford University |
1982 | 서울대학교 계측제어공학 석사 |
1980 | 서울대학교 전기공학 학사 |
Faced with the Winter of AI in early 1990's, Dr. Cha moved to the field of in-memory database management since he joined Seoul National University in 1992. To scale up his early university research, he founded a startup Transact In Memory, Inc., in Silicon Valley in 2002. The German software company SAP AG acquired this startup in late 2005. Since then, he led the conception and development of HANA, the world's first enterprise-scale in-memory database platform which finally became available in the global market in 2012.
Before HANA, disks and SSDs were used as the primary storage of large-scale enterprise database. Running big analytics and machine learning was very slow and real-time processing was impossible because the substantial volume of data must move from slow external storage to computer’s DRAM. Leveraging advances in DRAM and multi-core CPU, HANA changed the industry paradigm by using DRAM memory as the primary storage of database and providing innovative software mechanisms of fast database recovery and parallel real-time run of big analytics and machine learning inside the database platform.
Today, HANA is used by more than 17,000 companies in the world such as Apple, Walmart, Toyota, CVS Health Corporation, and Samsung Electronics. It is also available as a database service on Google, Amazon, and MS clouds. With the success of HANA, the era of in-memory computing has begun. This paradigm shift of using large memory in computing contributed to the growth of the Korean semiconductor industry.
Dr. Cha is a researcher, innovator, and entrepreneur who not only led the paradigm shift of an industry dominated by big companies but also created a new model of scaling academic research to market-disruptive innovation. With this experience, he founded a new Graduate School of Data Science at Seoul National University to educate future innovators with challenging spirit.
Invited Talks
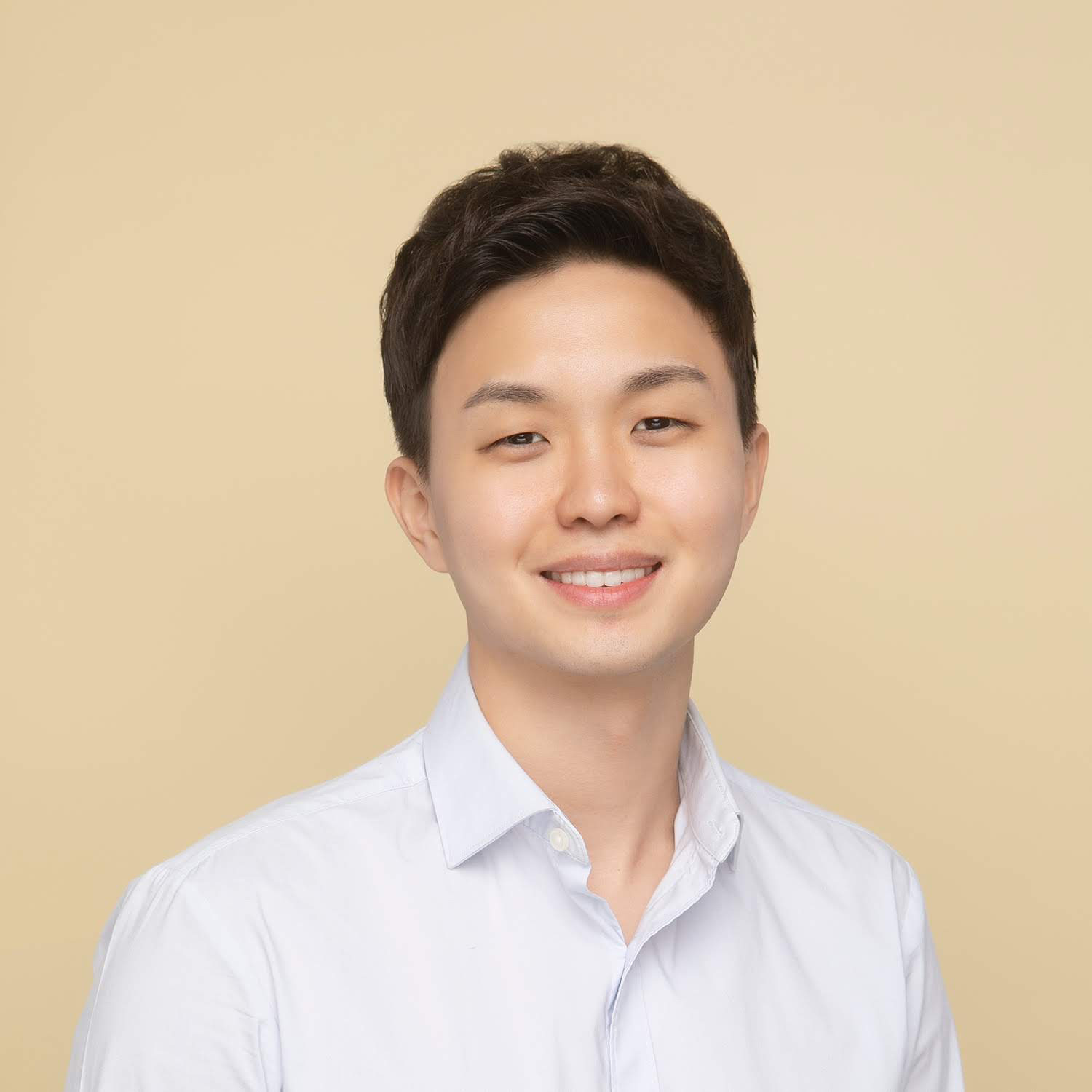
서민준교수
(KAIST)
Biography | |
---|---|
Minjoon Seo is an Assistant Professor at KAIST Graduate School of AI. He finished his Ph.D. at the University of Washington, advised by Hannaneh Hajishirzi and Ali Farhadi. His research interest is in natural language processing and machine learning, and in particular, how knowledge data can be encoded (e.g. external memory and language model), accessed (e.g. question answering and dialog), and produced (e.g. scientific reasoning). His study was supported by Facebook Fellowship and AI2 Key Scientific Challenges Award. He previously co-organized MRQA 2018, MRQA 2019 and RepL4NLP 2020. |
Controlling Knowledge in a Language Model (45분)
Large language models are known to be capable of storing a vast amount of the world knowledge. In this talk, I will discuss how we can add or update knowledge in a language model in a controlled manner without causing catastrophic forgetting. More specifically, I will present three recent projects on this topic (Continual Knowledge Learning, TemporalWiki, and Prompt Injection) and make an argument about what works and what is next.
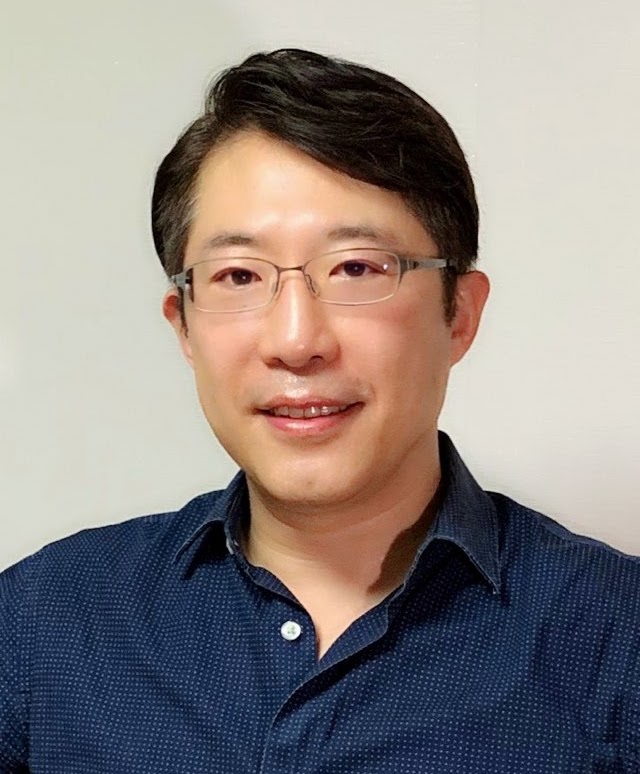
강재우 교수
(고려대학교)
Biography | |
---|---|
2021-현재 | ㈜아이젠사이언스 CEO/Founder |
2006-현재 | 고려대학교 컴퓨터학과 교수 |
2003-2006 | North Carolina State University 컴퓨터학과 조교수 |
2003 | University of Wisconsin-Madison 전산학 박사 |
2000-2001 | WISEngine Inc., CTO/Founder |
1997-1998 | Savera Systems Inc., 연구원 |
1996-1997 | AT&T Labs Research, 연구원 |
1996 | University of Colorado at Boulder 전산학 석사 |
1994 | 고려대학교 전산학 학사 |
Opportunities and Challenges in AI-Driven Drug Discovery and Development (45분)
The process of developing a new drug is long and difficult. In general, it takes more than 10 years of R&D period and 2.6 billion dollars of R&D costs before a single drug is approved. The bigger problem is that these costs are increasing every year. Some domain experts warn that if this trend continues, the pharmaceutical industry will become an industry with no ROI in a few years. In the pharmaceutical sector, there is a lot of interest in introducing AI to improve the chronic high cost and low efficiency structure of this industry. Can AI help solve this problem? AI has shown promise in many problems in the process of drug development, but I think there are still many areas lacking to show practical results. This talk outlines the drug development process from target identification, hit discovery, lead optimization, and clinical trial design, and discusses the contribution of AI at each stage and the challenges that current AI technology has to solve.
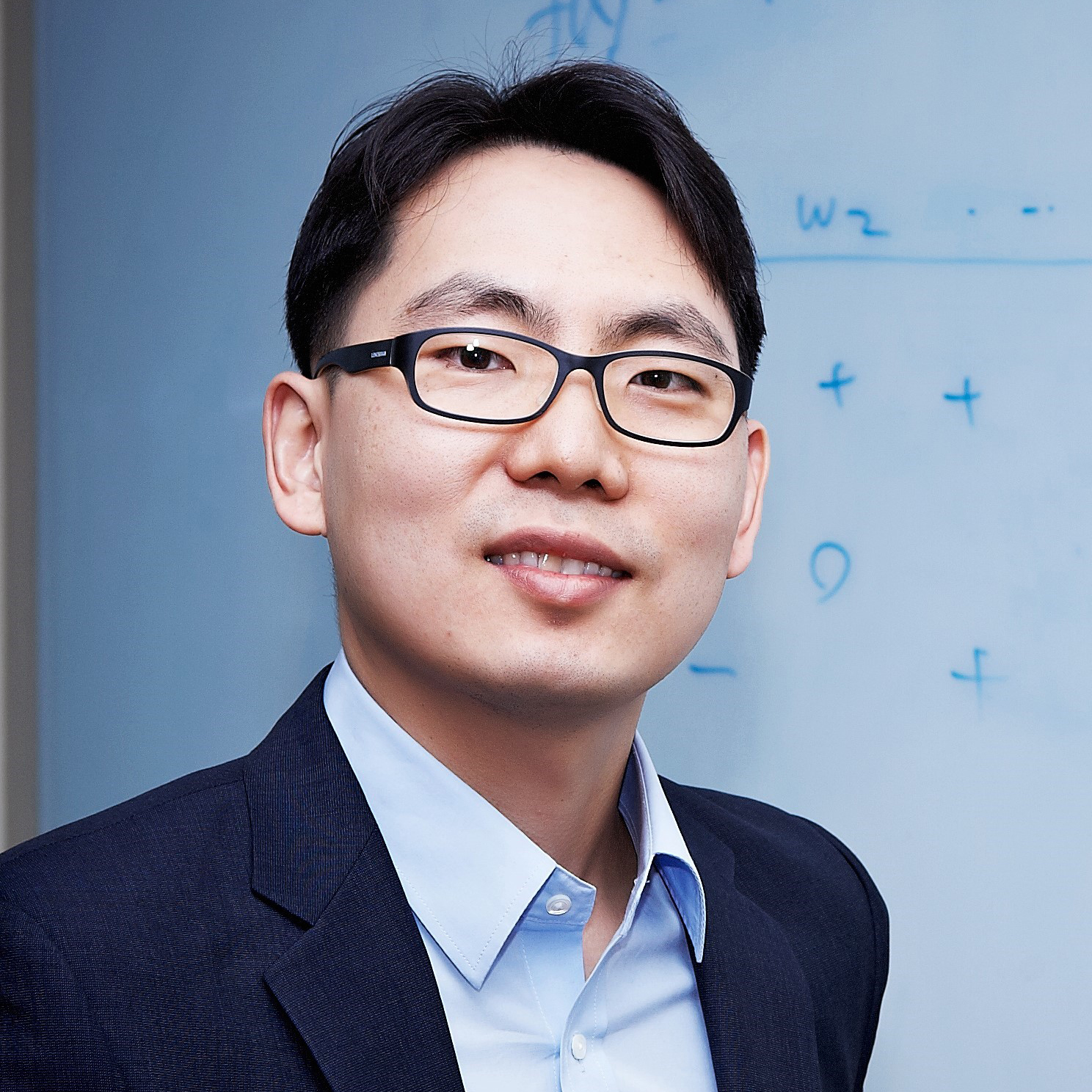
유환조 교수
(POSTECH)
Biography | |
---|---|
2021-현재 | (주)AI인사이트 CTO |
2019-현재 | (주)캐롯손해보험 사외이사 |
2016-2017 | 삼성전자 자문교수 |
2014-2015 | LG전자 자문교수 |
2008-현재 | POSTECH 컴퓨터공학/인공지능대학원 교수 |
2004-2007 | University of Iowa, 교수 |
2004 | University of Illinois at Urbana-Champaign, 전산학 박사 |
Recent advances in time-series analysis and anomaly detection (45분)
Unsupervised methods have been developed for anomaly detection because it is hard to obtain anomaly labels in practice. But, their performances are limited due to the absence of labels. This talk first introduces different approaches to overcome the limitation of anomaly detection using PU learning or weakly supervised learning. After that, this talk moves to the topic of time-series analysis. A critical challenge in time-series analysis is it is hard to get labels for segmentation or anomaly detection. I will present a technique for automatically segmenting time-series while maximizing classification performance. After that, I will present a time-series anomaly segmentation technique without segmentation labels. These works are published in AAAI2021 and ICCV2021.
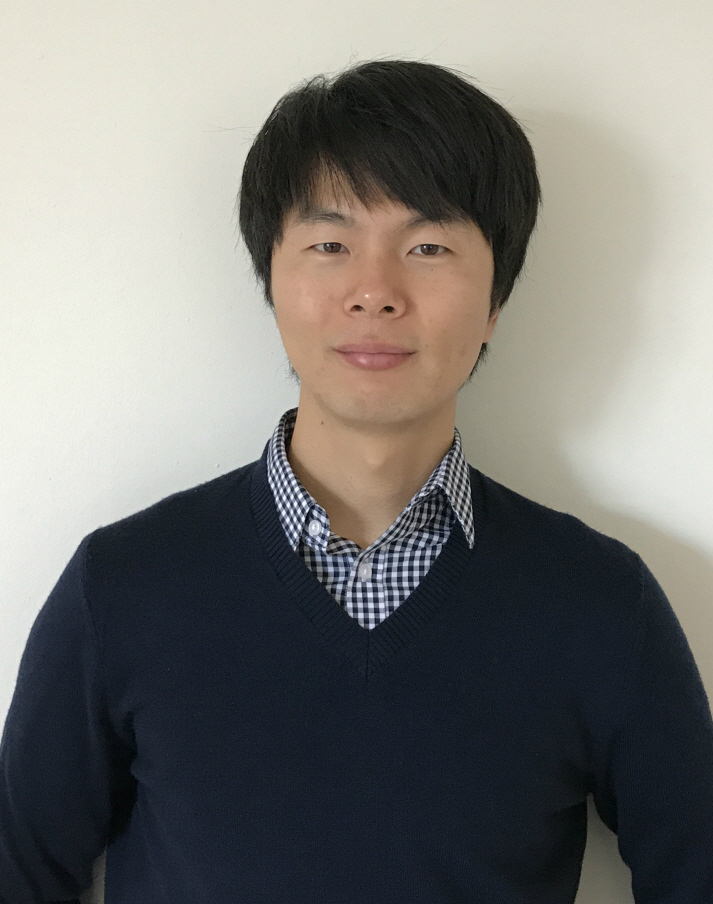
주한별 교수
(서울대학교)
Biography | |
---|---|
Hanbyul Joo is an assistant professor at Seoul National University (SNU) in the Department of Computer Science and Engineering. Before joining SNU, Hanbyul was a Research Scientist at Facebook AI Research (FAIR), Menlo Park. His research is at the intersection of computer vision, graphics, and machine learning, focusing on building a system to perceive and understand humans in 3D from visual input. Hanbyul received his PhD from the Robotics Institute at Carnegie Mellon University, and MS and BS from KAIST. Hanbyul’s research has been featured in various media outlets including Discovery, Reuters, NBC News, The Verge, and WIRED. He is a recipient of the Samsung Scholarship and the Best Student Paper Award in CVPR 2018. |
A Deep Dive into 2d and 3d Human Pose Estimation (45분)
Humans are born to move; we move for physical activities in our daily life and also for communication to convey our thoughts, emotions, and intentions through a concert of subtlest movements. However, despite advances in machine perception, machines are still unable to discern the subtle and momentary nuances of human behaviors that carry tremendous amounts of information and context. In this talk, I will give an overview on 2D and 3D human pose estimation fields. I will first begin with classic and recent trends in estimating human poses in 2D. I will also delve into the various directions in 3D human pose estimation.
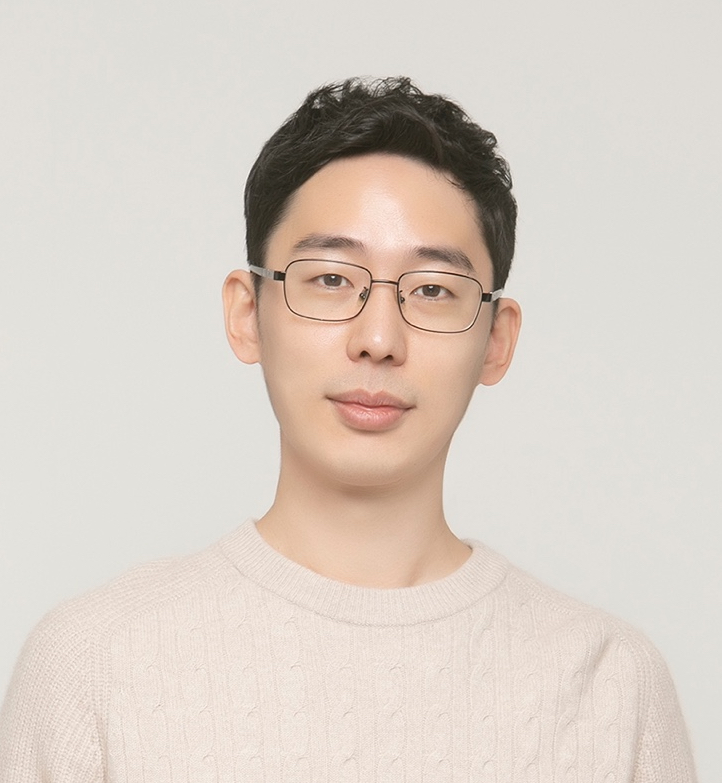
임재환 교수
(KAIST)
Biography | |
---|---|
He is an Associate Professor in the Kim Jaechul School of Artificial Intelligence at Korea Advanced Institute of Science and Technology (KAIST). Previously, he was an assistant professor at the University of Southern California (USC). Before that, he completed his PhD at Massachusetts Institute of Technology under the guidance of Professor Antonio Torralba, and also had a half-year long postdoc under Professor William Freeman and a year long postdoc under Professor Fei-Fei Li at Stanford University. He received his bachelor degree at the University of California - Berkeley, where he worked in the Computer Vision lab under the guidance of Professor Jitendra Malik. He also have spent time at Microsoft Research, Adobe Creative Technologies Lab, and Google. |
Towards Solving Complex Physical Tasks Via Learning Methods (45분)
Many robotics tasks, even seemingly simple procedural tasks like assembly and cleaning, require a continuous cycle of planning, learning, adapting and executing of diverse skills and sub-tasks. However, deep reinforcement learning algorithms developed for short-horizon tasks often fail on long-horizon tasks -- suffering from high-dimensionality of inputs and sample complexity. It is thus hard to scale and generalize learning agents to long-horizon complex tasks. To this end, my research centers on enabling autonomous agents to perform long-horizon, complex physical tasks. More specifically, I focus on how complex robotics tasks can be addressed by modularizing long-horizon tasks into multiple sub-tasks and skills; and address the following three main challenges: (1) how to generalize policies and reinforcement learning algorithms, (2) how to compose learned skills for a long-horizon task, and (3) how and what to learn from demonstrations. In this talk, I will describe some recents works from my lab and discuss future directions.
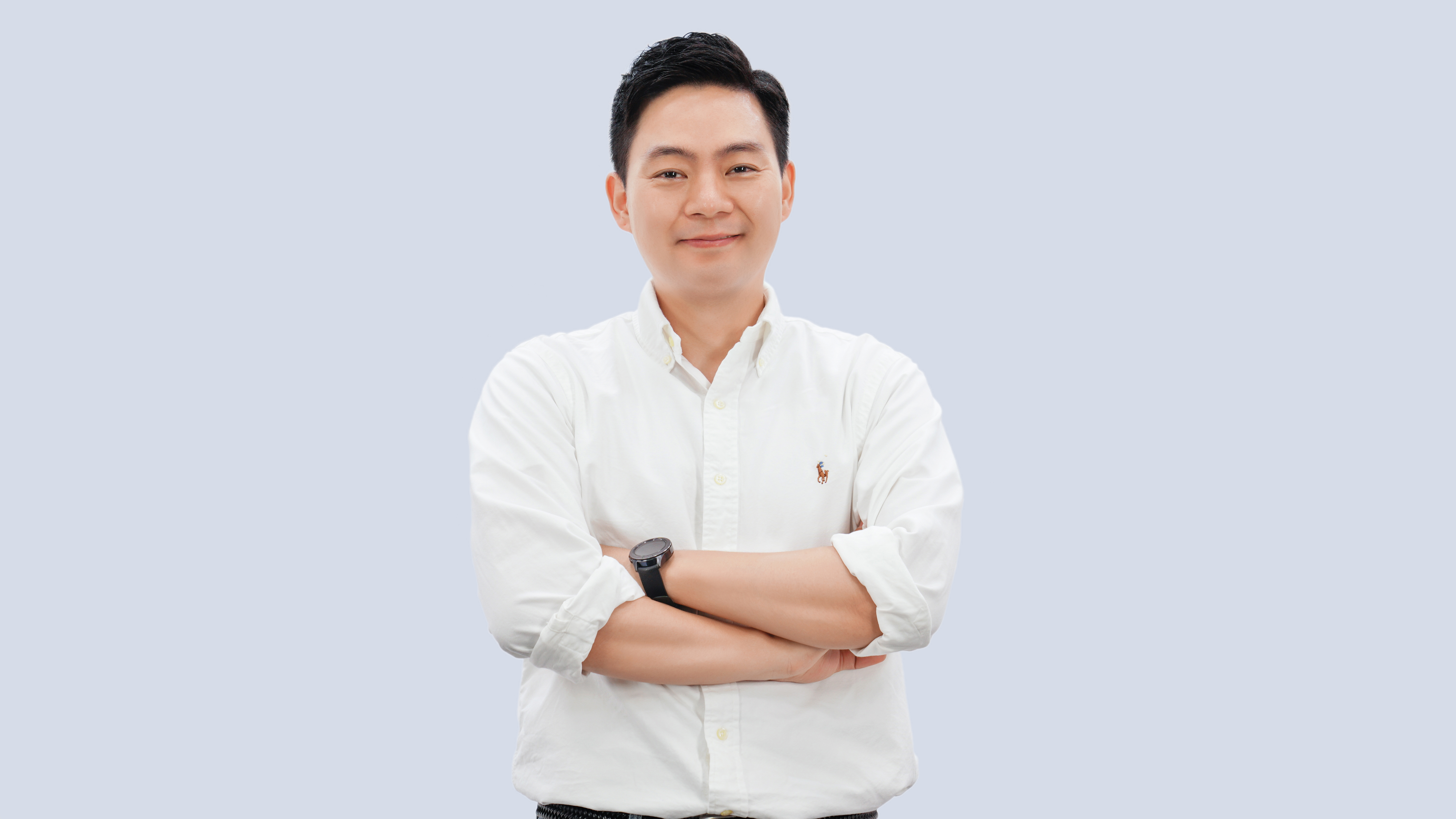
강남우 교수
(KAIST)
Biography | |
---|---|
2022–현재 | (주)나니아랩스 CEO |
2021–현재 | KAIST 조천식모빌리티대학원 조교수 |
2018–2021 | 숙명여자대학교 기계시스템학부 조교수 |
2014–2016 | University of Michigan, Mechanical Engineering, Research Fellow |
2007-2010 | 현대자동차, 연구원 |
2014 | Ph.D. Design Science, University of Michigan |
2007 | M.S. Technology and Management, Seoul National University |
2005 | B.S. Mechanical and Aerospace Engineering, Seoul National University |
AI Transformation for Product Development Process (45분)
제품 개발 프로세스의 AI Transformation이 일어나고 있습니다. Digital Transformation을 통해 구축된 가상제품개발 환경에서, AI의 도움으로 신제품 개발을 더 빠르고 더 스마트하게 할 수 있게 되었습니다. 본 강연은 데이터 기반의 딥러닝 기술과 물리 기반의 공학설계 기술이 융합된 "AI 기반의 제너레이티브 디자인" 기술을 소개합니다. 이 기술을 통해 AI가 스스로 제품 설계안을 (1)생성, (2)평가, (3)최적화, (4)추천할 수 있으며, 기존의 제품 개발 프로세스를 데이터 기반으로 혁신 할 수 있습니다. 본 기술이 적용된 다양한 모빌리티 제품 개발 사례들과, 이를 사업화한 설계 도메인 기반 AI 기업인 나니아랩스를 소개합니다.
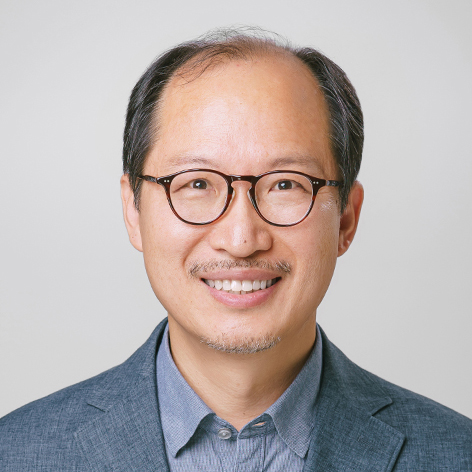
이지형 교수
(성균관대학교)
Biography | |
---|---|
2019-현재 | 성균관대학교 일반대학원 인공지능학과 학과장 |
2017-현재 | 성균관대학교 지능정보융합원 부원장 |
2017-현재 | 성균관대학교 일반대학원 데이터사이언스융합학과 학과장 |
2011-2013 | 성균관대학교 컴퓨터공학과 학과장 |
2000-2002 | Stanford Research Institute (SRI) International (미) (International Fellow) |
1996-1997 | AIO Microservice 사 (파견연구원) |
1995-1999 | Ph.D Korea Advanced Institute of Science and Technology |
1993-1995 | M.S Korea Advanced Institute of Science and Technology |
1989-1993 | B.S Korea Advanced Institute of Science and Technology |
Deep Semi-Supervised Learning (45분)
Recently, deep semi-supervised learning attracts a lot of attentions because supervised learning approaches usually require huge amounts of labeled samples. Semi-supervised learning approaches try to utilize unlabeled samples together with labeled samples. In this talk, recent advances in deep semi-supervised learning will be overviewed, and some important issues, such as deep semi-supervised learning with extremely scarce labeled samples and how to overcome the confirmation bias in deep semi-supervised learning, are discussed.
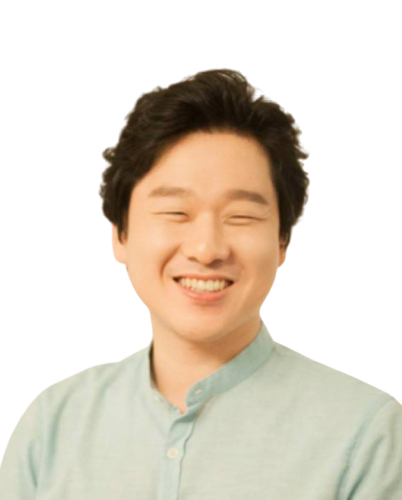
임성빈 교수
(UNIST)
Biography | |
---|---|
2020 - 현재 | UNIST, 산업공학과 및 AI 대학원, 조교수 |
2018-2019 | 카카오브레인, AI Scientist |
2017 | DeepBio, Research Engineer |
2016-2017 | 삼성화재, Data Scientist |
2016 | 고려대학과 수학과 박사 |
2010 | 고려대학교 수학과 및 정치외교학과 학사 |
Neural Bootstrapper and its Applications to Neural Processes (45분)
Bootstrapping 은 통계학과 머신러닝에서 uncertainty quantification 에 많이 사용되는 방법론입니다. 그러나 기존 bootstrap sampling 방식은 neural network 의 예측 분포를 학습할 때 지나치게 많은 연산량과 메모리를 요구하며, 데이터 사이즈가 커지는 경우 일부 데이터를 관찰하지 못하는 문제가 발생하게 만듭니다. 이러한 문제들을 해결하기 위해 제안된 Neural Bootstrapper (NeuBoots) 방법론과, Neural Process 를 bootstrapping 하는 응용 방법도 같이 소개합니다.