11월 22일 (수) | |||
10:00 - 12:00 |
|
||
14:00 - 16:00 |
|
11월 23일 (목) | |||
10:00 - 12:00 |
|
||
14:00 - 16:00 |
|
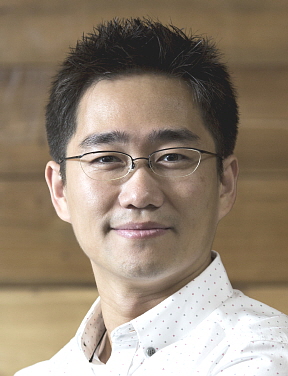
주재걸 교수
(KAIST 김재철AI대학원)
Biography | |
---|---|
2020.03~현재 | KAIST 김재철AI대학원 부교수 |
2019.09 – 2020.02 | 고려대학교 인공지능학과 부교수 |
2015.03 – 2019.08 | 고려대학교 컴퓨터학과 조교수 |
2001 | 학사, 서울대학교 전기공학부 |
2009 | 석사, Electrical and Computer Engineering, Georgia Tech |
2013 | 박사, Computational Science and Engineering, Georgia Tech |
3D-aware Image Generation and Editing Approaches and Applications (120분)
Recently, 3D-aware image generation and editing techniques has been actively studied, significantly advancing generated image quality and speed. In this talk, I will cover various different problem settings and their relevant approaches, along with real-world applications.
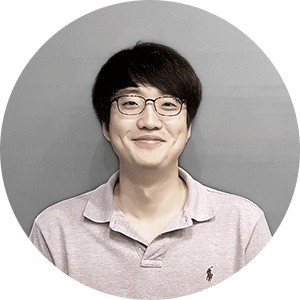
김세훈 그룹장
(카카오브레인)
Biography | |
---|---|
2020-현재 | 카카오브레인 AI Researcher |
2017-2019 | AITRICS 연구팀장 |
2018 | POSTECH 컴퓨터공학 박사 |
2012 | MSRA, MSR 연구 인턴 |
2009 | POSTECH 컴퓨터공학 학사 |
Diffusion Generative Models for Conditional Image Generation (120분)
Diffusion Generative Models (DGM) are iterative procedures that progressively refine corrupted samples from uninformative priors, typically the standard Gaussian distribution. This class of generative models explicitly aims to maximize the likelihood of the data, effectively encompassing all modes within the data distribution. As a result, DGMs tend to generate more diverse samples compared to implicit generative models. This presentation is divided into three parts: the formulation of diffusion processes, the famous models focusing on conditional generative models, and our attempts to contribute to this research direction. Firstly, I will cover the basic framework for training and sampling generative diffusion processes, without delving into mathematical details. Subsequently, I will describe some details of the formulation of DGMs from the perspective of latent variable models (Ho et al., 2020) and stochastic differential equations (Song et al., 2021). Secondly, I will discuss the extension of the diffusion framework to handle high-resolution images, covering the core concepts of Cascade Diffusion Models (CDM) and Latent Diffusion Models (LDM). Additionally, I will explain how to integrate condition information to develop conditional generative models, utilizing techniques like cross-attention, modulation, and classifier-free guidance. Finally, I will conclude by outlining our Karlo projection, which is a cascade diffusion model with a mixture of experts that has been designed for efficiency. Its super-resolution module is trained using a hybrid objective, which combines likelihood-based and adversarial losses. This approach ensures that the model can produce high-quality outputs while maintaining a fast inference time.
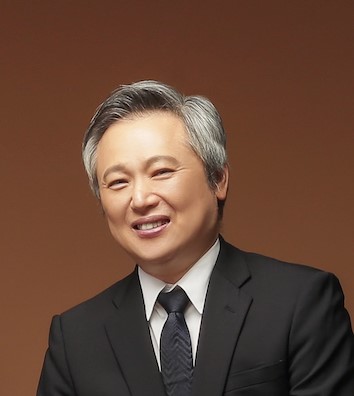
최승진 소장
(Intellicode)
Biography | |
---|---|
2022-현재 | 연구소장, Intellicode |
2019-2021 | CTO, BARO AI & 상임고문, BARO AI Academy |
2001-2019 | POSTECH 컴퓨터공학과 교수 |
2019-2021 | 정보과학회 인공지능소사이어티 회장 |
2018 | 삼성전자 종합기술원 자문교수 |
2017-2018 | 삼성리서치 AI센터 자문교수 |
2016-2017 | 신한카드 빅데이터센터 자문교수 |
2014-2016 | 정보과학회 머신러닝연구회 초대위원장 |
Score-based Generative Models (120분)
Generative models have gained significant attention in machine learning for their ability to generate realistic data samples, opening the door to various applications like image synthesis, text generation, and more. Score-based generative models have recently emerged as a promising approach to data generation, outperforming GANs in the task of image generation. In this talk, I provide a comprehensive overview of score-based generative models. Commencing with the fundamental concept of score matching, we explore how to learn unnormalized statistical models from data by estimating the gradient field of the logarithmic data distribution. Additionally, I introduce two scalable score matching methods such as denoising score matching and sliced score matching. In the context of generative models, I delve into the noise conditional score networks, a novel approach enabling the learning of data scores at multiple noise levels. I also elucidate the annealed Langevin Monte Carlo as a mechanism for sample generation. Furthermore, I discuss the extension of this model to accommodate infinite noise levels, wherein stochastic differential equations (SDEs) play a pivotal role in modeling trajectories from data to noise. The reverse-time SDEs are leveraged for sample generation from noise. This talk offers a thorough overview of score-based generative models, shedding light on their fundamental principles.
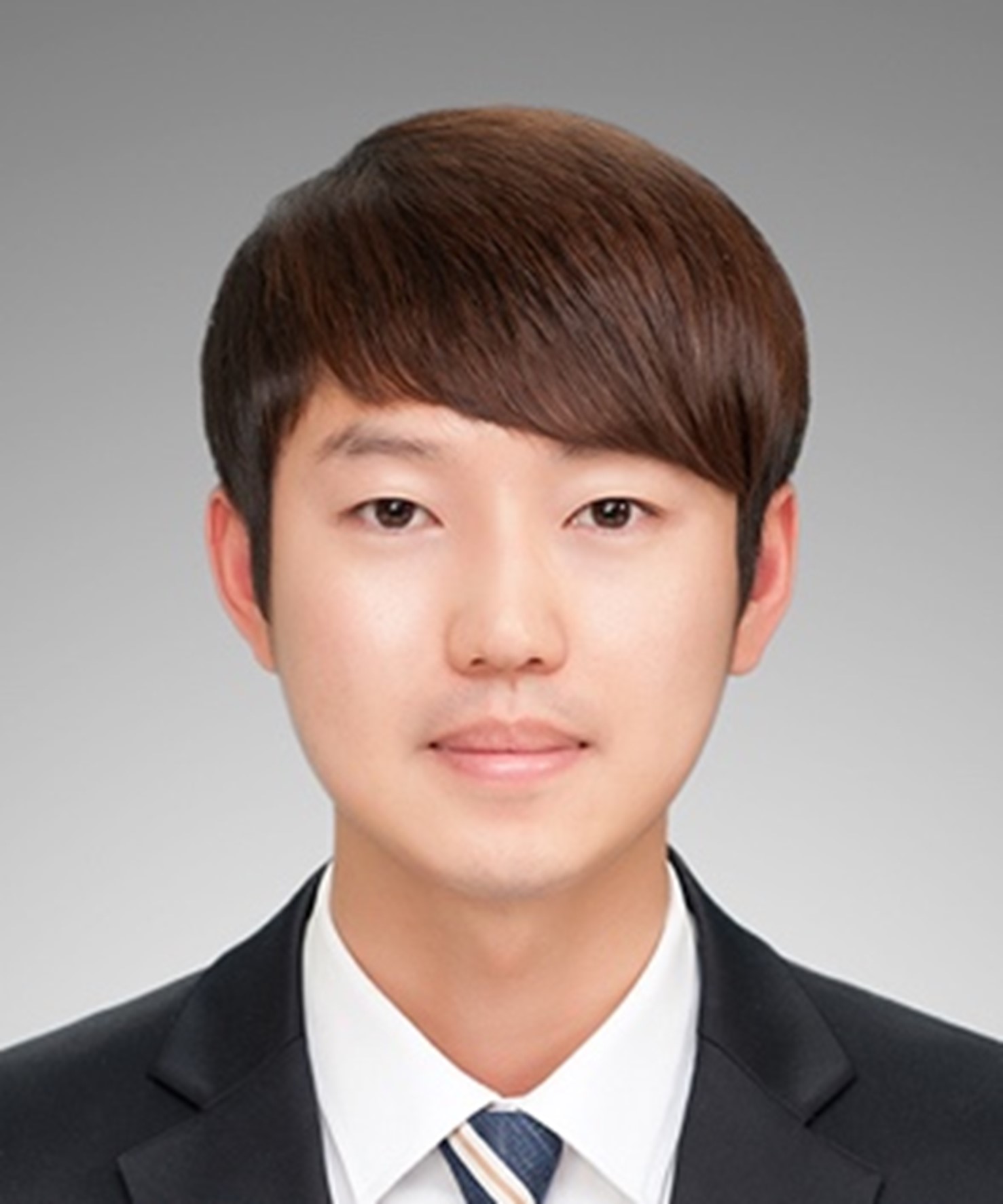
김승룡 교수
(Intellicode)
Biography | |
---|---|
2018 | 연세대학교 전기전자공학과 박사취득 |
2018-2019 | 연세대학교 전기전자공학과 박사후 연구원 |
2019-2020 | 스위스 EPFL 박사후 연구원 |
2020 | 고려대학교 컴퓨터학과 조교수 |
Text-to-3D Generation Using 2D Diffusion Model (120분)
Text를 입력으로 받아 3차원 모델을 만드는 기술은 최근에 Diffusion Model과 Neural Radiance Fields (NeRF)의 성공에 힘입어 실제적인 적용이 가능한 수준의 결과들이 공개되고 있다. 하지만 이러한 기존의 기술은 2차원 Diffusion Model에만 의존하다보니 생성된 3차원 모델이 View-inconsistent 한 결과를 자주 보여준다. 본 세미나에서는 이러한 View-inconsistency 문제를 해결하기 위한 연구들을 소개하고 향후 방향성에 대해서 논의해보고자 한다.