특별 강연
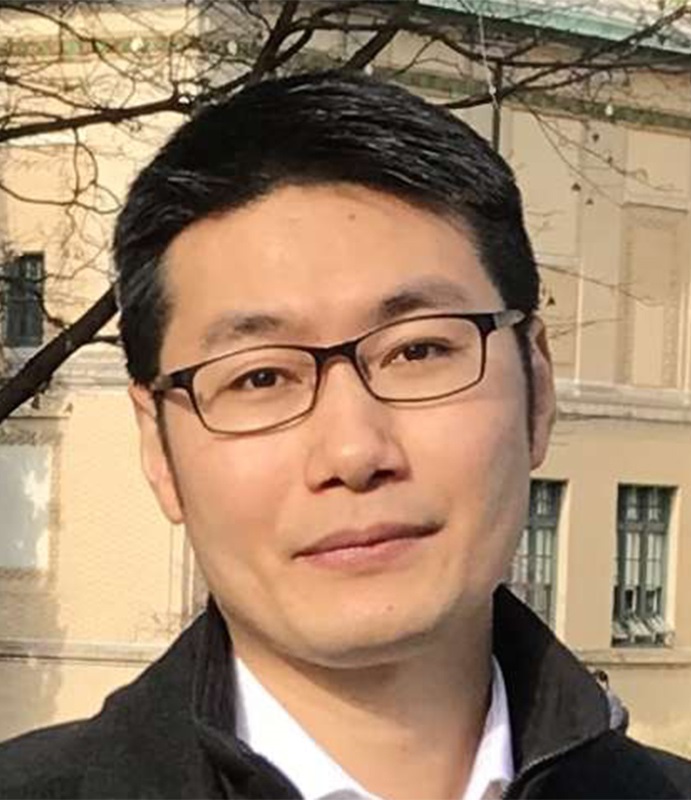
Causality and Learning
Kun Zhang(Carnegie Mellon University)
Does smoking cause cancer? Can we find the causal direction between two variables by analyzing their observed values? In our daily life and science, people often attempt to answer such causal questions, for the purpose of understanding and manipulating systems properly. On the other hand, we are also often concerned with how to do machine learning in complex environments, such as learning under data heterogeneity. For instance, how can we make optimal predictions in non-stationary environments? In the past decades, interesting advances were made in fields including machine learning, statistics, and philosophy for tackling long-standing causality problems, including how to discover causal knowledge from purely observational data and how to infer the effect of interventions using such data. Furthermore, it has recently been shown that causal information can facilitate understanding and solving various machine learning problems, including transfer learning and semi-supervised learning. This tutorial reviews essential concepts in causality studies and is focused on how to learn causal relations from observation data and why and how the causal perspective helps in machine learning and other tasks. A number of open problems will also be posed in the tutorial.
초청강연
7월 25일(목요일) 초청강연 | |
11:00 - 12:30 | 김건희(서울대학교): Recent Advances in Abstractive Summarization and Audio Captioning |
최종현(GIST): Visual Recognition with Weak Supervision | |
16:00 - 17:30 | 김기응(KAIST): Adversarial Approaches to Imitation Learning |
강재우(고려대학교):AI Medicine: Data-driven Drug Discovery | |
7월 26일(금요일) 초청강연 | |
09:20 - 10:50 | 이슬(서울대학교):Interpretable Multi-dimensional Data Analysis with Tensor Mining |
조민수(POSTECH): Relational Knowledge Distillation | |
11:10 - 12:40 | 이상완(KAIST): Neuroscience-inspired AI |
김선(서울대학교): Decomposing tumor into distinct cell groups using HMM and EM | |
14:10 - 15:40 | 최재식(UNIST): Recent Advances in Interpretable and Explainable Artificial Intelligence |
양은호(KAIST): Advanced optimizers for deep learning | |
16:00 - 17:30 | 윤성로(서울대학교): Deep Generative Models for Practical Applications of Deep Learning |
황성주(KAIST): Learning to Balance: Bayesian Meta-Learning for Imbalanced and Out-of-distribution Tasks |
연사 및 세션소개
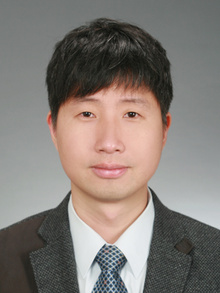
Recent Advances in Abstractive Summarization and Audio Captioning
김건희(서울대학교)
In this talk, I will introduce two recent papers about NLP from Vision and Learning Lab of Seoul National University. First, I discuss about our work on abstractive summarization in which we address the problem in two directions: collecting a novel dataset from the online discussion forum Reddit and proposing a new model based on multi-level memory networks. Second, we present our work that explores the problem of audio captioning: generating natural language description for any kind of audio in the wild, which has been surprisingly unexplored in previous research. We not only contribute a large-scale dataset of about 46K audio clips to human-written text pairs collected via crowdsourcing but also propose two novel components that help improve audio captioning performance of attention-based neural models.
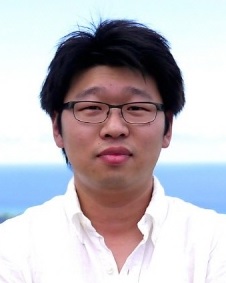
Visual Recognition with Weak Supervision
최종현(GIST)
Curating datasets for visual recognition (e.g., image classification) is expensive and visual recognition with weak supervision suffers from poor accuracy. To improve the classification accuracy, I will discuss a few ideas to learn image classification models with weakly supervised data. The ideas include 1) using semi-supervised learning with unlabeled data, 2) using iterative human feedback and 3) using geometric prior. Further, I will discuss about emerging sub-areas for visual recognition research.
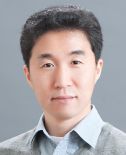
Adversarial Approaches to Imitation Learning
김기응(KAIST)
Imitation learning is a machine learning problem where we make the decision-making agent learn to imitate the behavior of an expert. Recent approaches to this problem adopts adversarial optimization frameworks that have shown to successfully imitate behaviors in high-dimensional and complex tasks, leveraging the power of deep reinforcement learning algorithms and generative adversarial networks. I will cover how these ideas emerged from the classical treatment of the problem, and highlight our recent work on imitation learning.
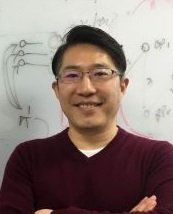
AI Medicine: Data-driven Drug Discovery
강재우(고려대학교)
AI plays an increasingly important role in precision medicine and drug discovery. In this talk, I will introduce some of our recent efforts in AI-driven precision medicine and drug discovery, primarily in the context of our experiences in competing in international precision medicine competitions called DREAM Challenges. DREAM Challenges are a series of competitions, aiming to solve challenging problems in precision medicine, through a collaborative community effort. The first challenge we participated was the Astra-Zeneca Sanger Drug Combination DREAM challenge. The participants were expected to design a machine learning model to predict the synergistic combination of cancer drugs for each individual patient. We used the genomic and transcriptional features of patients and the chemical properties of drugs to predict the synergy. Our model was ranked at the second place in the competition. The second competition we participated was the NCI-CPTAC Proteogenomics DREAM Challenge. In the challenge, the participants were asked to build a machine learning model to predict the protein abundance using the abundances of other proteins or the copy number and the expression level of the given protein. We built a prediction model based on a collaborative filtering algorithm. Our model achieved the first place in the competition. Finally, I will introduce the Multi-targeting Drug DREAM Challenge. The challenge asked the participants to submit a list of drug candidates that can bind to a given set of targets and at the same time can avoid a given set of non-targets. We built Siamese Neural Networks called ReSimNet to predict the transcriptional response phenotype similarities between drugs. The drug candidates selected using our ReSimNet was determined as the winner of the competition.
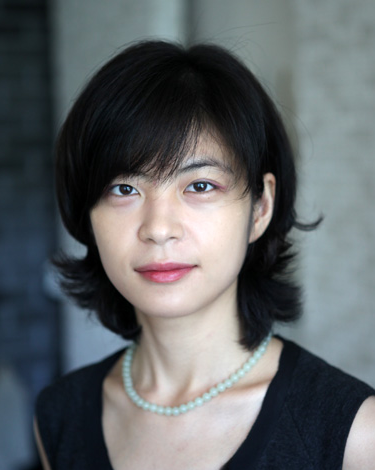
Interpretable Multi-dimensional Data Analysis with Tensor Mining
이슬(서울대학교)
Many real-world data can be represented as a tensor, i.e., a multi-dimensional array. Tensors can be analyzed using tensor decomposition methods such as Tucker decomposition that decomposes an input tensor into a core tensor and factor matrices. Resulting core tensor and factor matrices are often dense and complex. Thus, information from the data can be acquired only after additional post-analysis steps, such as clustering. How can we devise tensor factorization methods such that the results are directly interpretable? I will introduce three interpretable methods GIFT, CTD, and VeST which I recently proposed, and show how their results are interpretable. GIFT (Guided and Interpretable Factorization of Tensors) utilizes prior grouping information of input features to focus the latent feature learning of a factor matrix around the members of the groups. CTD (Compact Tensor Decomposition) utilizes already sparse and interpretable features of input tensor in the construction of the factor matrix. VeST (Very Sparse Tucker factorization) decomposes the input tensor to sparse core tensor and factor matrices where the sparsity of the results enhances interpretability. The methods are successfully applied on, bio-clinical data, network data, and rating data, respectively.
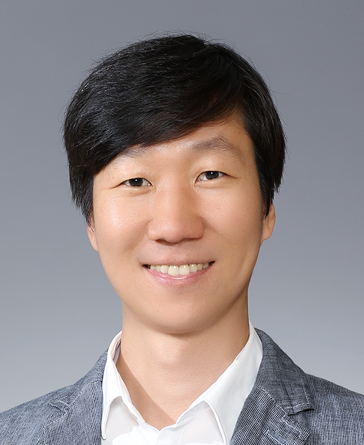
Relating Konwledge Distillation
조민수(POSTECH)
Knowledge distillation aims at transferring ``knowledge'' acquired in one model (teacher, e.g., a cumbersome neural network) to another model (student, e.g., a shallow neural network) that is typically smaller. Previous approaches can be expressed as a form of training the student with output activations of data examples represented by the teacher. In this work we revisit KD from a perspective of the linguistic structuralism, which focuses on structural relations in a semiological system. Saussure's concept of the relational identity of signs is at the heart of structuralist theory; ``In a language, as in every other semiological system, what distinguishes a sign is what constitutes it''. In this perspective, the meaning of a sign depends on its relations with other signs within the system; a sign has no absolute meaning independent of the context. From this perspective, we introduce a novel approach, dubbed relational knowledge distillation (Relational KD), that transfers relations among data examples represented by the teacher. As concrete realizations of Relational KD, we propose distance-wise and angle-wise distillation losses that penalize structural differences in relations. Experiments conducted on different benchmark tasks show that the Relational KD improves the performance of the educated student networks with a significant margin, and even outperforms the teacher's performance.
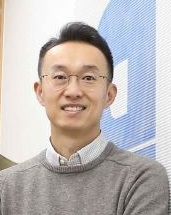
Neuroscience-Insipired AI
이상완(KAIST)
Neural networks have evolved from data-driven types into a self-play type, owing to a combination of deep learning and reinforcement learning (RL). This class of algorithms has demonstrated an ability to succeed in a few arduous tasks, emerging as a general framework for decision making in neuroscience and robotics. That being said, there is still a plentitude of brain’s advanced capabilities that the state-of-the-art AI hasn’t acquired yet. This talk introduces our team’s research topics to interface neuroscience and AI, aiming to both understand and engineer human RL. The first part of the talk focuses on the prefrontal-striatal circuitry for RL. By using a combination of model-based experimental design and computational modelling, I will discuss the structure and dynamics of prefrontal-striatal network for meta RL. These evidences accumulate to suggest a theoretical idea about how the meta RL handles technological challenges: performance-efficiency, speed-accuracy, and exploration-exploitation. The second part of the talk outlines more pragmatic approaches to implanting this ability into AI. A detailed insight into these issues not only permits advances in machine learning, but also helps us understand the nature of human intelligence on a deeper level.
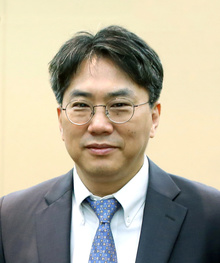
Decomposing Tumor into Distinct Cell Groups using HMM and EM
김선(서울대학교)
Characterizing cancer subclones is crucial for the ultimate conquest of cancer. Thus, a number of bioinformatics tools have been developed to infer heterogeneous tumor populations based on genomic signatures such as mutations and copy number variations. However, despite accumulating evidence for the significance of global DNA methylation reprogramming in certain cancer types including myeloid malignancies, none of the bioinformatic tools are designed to exploit subclonally reprogrammed methylation patterns to reveal constituent populations of a tumor. In accordance with the notion of global methylation reprogramming, our preliminary observations on acute myeloid leukemia samples implied the existence of subclonally-occurring focal methylation aberrance throughout the genome. We present PRISM, a tool for inferring the composition of epigenetically distinct subclones of a tumor solely from methylation patterns obtained by reduced representation bisulfite sequencing. PRISM adopts DNA methyltransferase 1 (DNMT1)-like hidden Markov model-based \textit{in silico} proofreading for the correction of erroneous methylation patterns. With error-corrected methylation patterns, PRISM focuses on a short individual genomic region harboring dichotomous patterns that can be split into fully methylated and unmethylated patterns. Frequencies of such two patterns form a sufficient statistic for subclonal abundance. A set of statistics collected from each genomic region is modeled with a beta-binomial mixture. Fitting the mixture with expectation-maximization algorithm finally provides inferred composition of subclones. Applying PRISM for two acute myeloid leukemia samples, we demonstrate that PRISM could infer the evolutionary history of malignant samples from an epigenetic point of view. PRISM is freely available on GitHub (https://github.com/dohlee/python-prism).
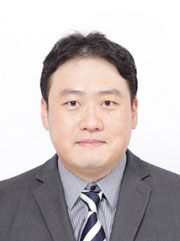
Recent Advances in Interpretable and Explainable Machine Learning Models
최재식(UNIST)
As many complex AI systems are deloveped and used in our daily lives. It becomes important to interpret and explain the decision of complex AI systems. In this talk, I will overview various methods and perspective in explainable Artificial Intelligence (XAI) methods. Topics include interpretable deep neural networks models, Bayesian model compositions, and model agnostic approaches. Furthermore, I will present a new GP model which naturally handles multiple time series by placing an Indian Buffet Process (IBP) prior on the presence of shared kernels. Our selective covariance structure decomposition allows exploiting shared parameters over a set of multiple, selected time series. We also investigate the well-definedness of the models when infinite latent components are introduced. We present a pragmatic search algorithm which explores a larger structure space efficiently. Experiments conducted on five real-world data sets demonstrate that our new model outperforms existing methods in term of structure discoveries and predictive performances.
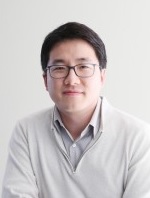
Advanced optimizers for deep learning
양은호(KAIST)
In this talk, we discuss some recent advances in optimizers for deep models. First, we briefly consider how we can efficiently learn sparse structures of deep models, which is useful for identifying relevant features or sparsifying network structures. We also skim over some theoretical findings on simple trimming L1 approach in this direction. For the remaining part of the talk, we focus on adaptive gradient approaches such as ADAGRAD, RMSPROP, ADAM and further recent extensions that automatically adjust the learning rate on a per-feature basis. All algorithms in this rich class have adopted diagonal matrix adaptation, due to the prohibitive computational burden of manipulating full matrices in high-dimensions. We look at some simple but powerful solution that can effectively utilize structural characteristics of deep learning architectures, and significantly improve convergence and out-of-sample generalization theoretically and empirically.
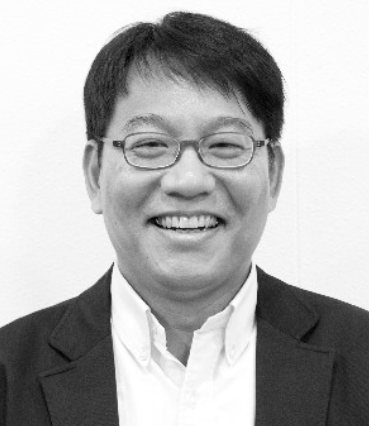
Deep Generative Models for Practical Applications of Deep Learning
윤성로(서울대학교)
In the first part, I will present a generative adversarial network (GAN) framework for handling practical classification problems. Most deep learning classification studies assume clean data. However, when dealing with the real world data, we encounter three problems such as 1) missing data, 2) class imbalance, and 3) missing label problems. Various preprocessing techniques have been proposed to mitigate one of these problems, but an algorithm that assumes and resolves all three problems together has not been proposed yet. I will describe HexaGAN, a generative adversarial network framework that shows promising classification performance for all three problems. The second part of my talk will cover the topic of raw audio synthesis. Most modern text-to-speech architectures use a WaveNet vocoder for synthesizing high-fidelity waveform audio, but there have been limitations, such as high inference time, in its practical application due to its ancestral sampling scheme. The recently suggested Parallel WaveNet and ClariNet have achieved real-time audio synthesis capability by incorporating inverse autoregressive flow for parallel sampling. However, these approaches require a two-stage training pipeline with a well-trained teacher network and can only produce natural sound by using probability distillation along with auxiliary loss terms. In this talk, I will introduce FloWaveNet, a flow-based generative model for raw audio synthesis. FloWaveNet requires only a single-stage training procedure and a single maximum likelihood loss, without any additional auxiliary terms, and it is inherently parallel due to the characteristics of generative flow.
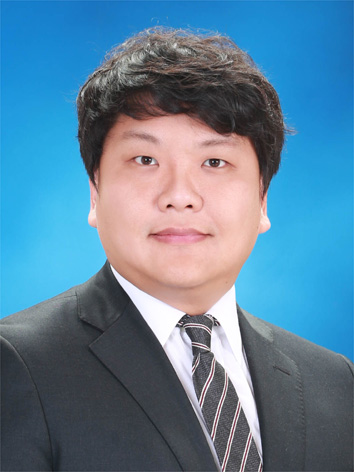
Learning to Balance: Bayesian Meta-Learning for Imbalanced and Out-of-distribution Tasks
황성주(KAIST)
While tasks could come with varying number of instances in realistic settings, the existing meta-learning approaches for few-shot classification assume even task distributions where the number of instances for each task and class are fixed. Due to such restriction, they learn to equally utilize the meta-knowledge across all the tasks, even when the number of instances per task and class largely varies. Moreover, they do not consider distributional difference in unseen tasks at the meta-test time, on which the meta-knowledge may have varying degree of usefulness depending on the task relatedness. To overcome these limitations, we propose a novel meta-learning model that adaptively balances the effect of the meta-learning and task-specific learning, and also class-specific learning within each task. Through the learning of the balancing variables, we can decide whether to obtain a solution close to the initial parameter or far from it. We formulate this objective into a Bayesian inference framework and solve it using variational inference. Our Bayesian Task-Adaptive Meta-Learning (Bayesian-TAML) significantly outperforms existing meta-learning approaches on benchmark datasets for both few-shot and realistic class- and task-imbalanced datasets, with especially higher gains on the latter.